The 5 perimeters of "AI"
Instead of projecting the future of AI and speculating on how fast the technological roadmap will advance (or stall), it's far more effective to focus on understanding the specific perimeters in which AI can reliably operate today.
In the same way that I was discussing back in 2019 with autonomous vehicles, AI would be better understood not according to Moore's Law and how fast we expect it to reach human-level intelligence, but rather in which perimeters it's currently adequate to use.
Years ago, autonomous vehicles were already pretty safe on a private track, where the environment was fully understood and predictable. On highways, expectations rise — the system must handle speed, predictable flows, and occasional incidents with minimal error. And then in a dense city center, with pedestrians, roadworks, and chaotic drivers, the tolerance for error becomes nearly zero, while self-driving cars can be rapidly overwhelmed by unpredictable situations.
AI systems follow the same logic.
Their perimeter of use defines the level of trust and the acceptable error margin, not the abstract notion of "AI intelligence" itself. Pretty much the current state of AI makes it OK to use if you're ready to accept 80% reliability. More and you open yourself for sore disappointment, if not plain danger. This straightforward logic will immediately change the way you see and understand how you should deal with AI.
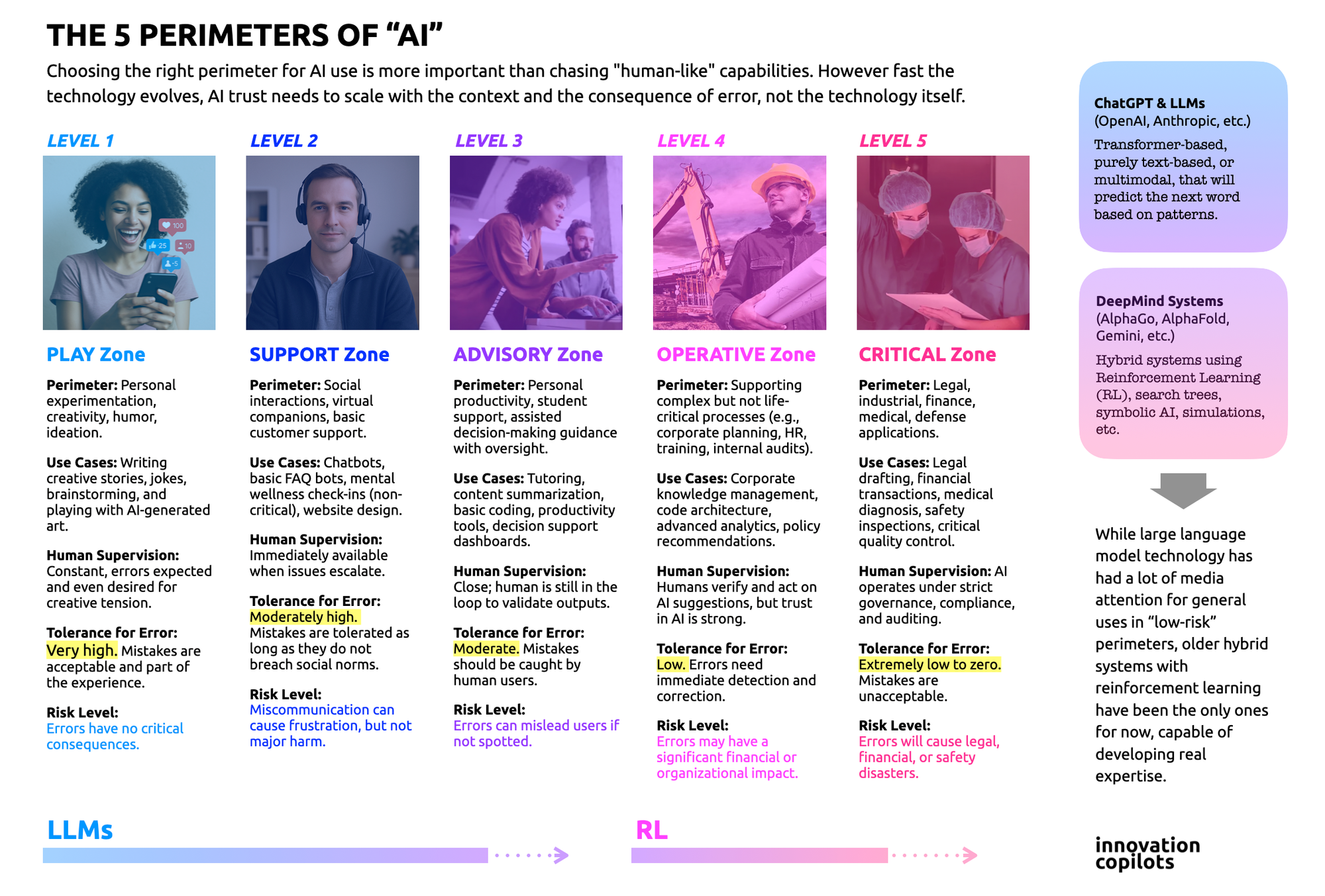
In playful or creative tasks (Level 1), errors are inconsequential — if not part of the charm.
When providing basic support (Level 2), mistakes may lead to some level of frustration, which remains acceptable, if annoying.
As we move into advisory or decision-support contexts (Level 3), AI is expected to assist and be right around 80% of the time, helping to offload tedious and repetitive tasks that add little value. However, human validation of outputs remains essential.
At Level 4, where AI becomes a trusted partner in complex tasks, the tolerance for error shrinks dramatically. LLMs (like GPT-style AI) should largely be avoided here, while other specialized technologies (e.g., DeepMind, AlphaGo) can prove powerful for dedicated, vertical problem-solving scenarios.
Finally, in regulated, high-stakes environments (Level 5), AI must operate with industrial-grade precision, domain-level expertise, and full accountability. This is unlikely to ever be a space for LLMs, which, by design, will always carry a certain degree of hallucination risk.
Case and point:
Directly put, Plaintiff's use of AI affirmatively misled me," Judge Wilner wrote in a May 5 order. "I read their brief, was persuaded (or at least intrigued) by the authorities that they cited, and looked up the decisions to learn more about them—only to find that they didn't exist. That's scary. It almost led to the scarier outcome (from my perspective) of including those bogus materials in a judicial order. Strong deterrence is needed to make sure that attorneys don't succumb to this easy shortcut." - Judge admits nearly being persuaded by AI hallucinations in court filing
Ultimately, the trust we place in AI systems must be shaped by the context and perimeter in which they operate — not by their perceived technical sophistication, nor by wishful projections of how fast they might become more reliable.