Are you working on 80%-OK or 99%-OK problems?
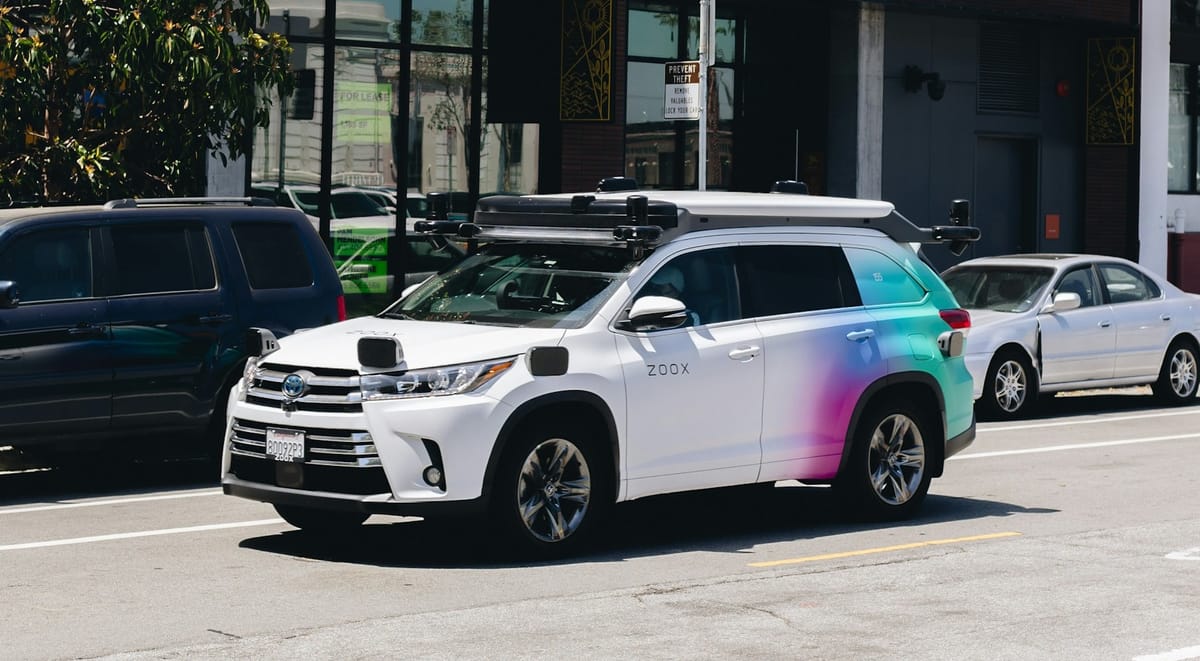
Another concept I’ve been mulling over this summer is the distinction innovators face between problems that can afford to be mostly solved (80%-OK) before delivering their core value and those that demand near perfection (99%-OK).
Take self-driving cars, for instance. Back in 2018, I predicted they’d be street-legal by 2020. Technically, I was right—there were indeed self-driving cars on the streets in 2020, but only as small-scale proofs of concept. It turns out self-driving is a 99%-OK problem: until safety is nearly flawless, the technology won’t break into the core market or achieve large-scale adoption.
On the flip side, the current iteration of AI, particularly large-language models, is more of an 80%-OK problem. Sure, they might hallucinate or produce factual errors if you let them write your PhD thesis. Still, for emails, blog posts (yikes), business reports, or most professional writing, they do a fine job—hence the explosive mass adoption of late.
I’m not sure if anyone has articulated these two categories of problems better (or at all), but I’m curious. It’s becoming clear to me that making this distinction early on in your innovation project can be game-changing—not just for strategy but also when considering the possibility of pivoting to another market. For instance, if you were a startup needing to pivot away from street-legal self-driving because 99%-OK problems are too long and hard to crack, you might shift to more manageable 80%-OK problems by targeting markets where the self-driving perimeters are easier to handle.